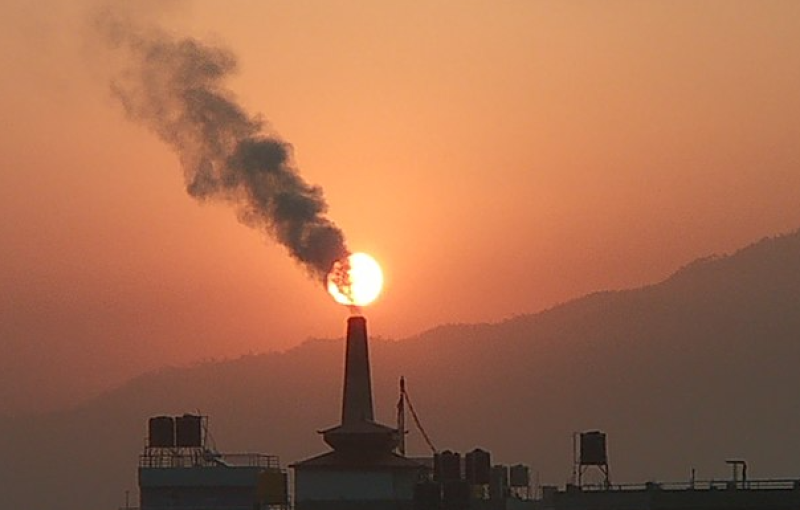
The Science of Predicting and Preventing Air Pollution
by Jon Scaccia December 3, 2024Imagine stepping outside for a morning jog, only to find the air thick with smog. It’s not just unpleasant—it’s dangerous. Air pollution is a silent killer, contributing to millions of deaths annually and impacting ecosystems worldwide. While the problem is enormous, researchers are turning to advanced technologies like machine learning and deep learning to predict and mitigate pollution’s impact. How are these tools transforming our fight for cleaner air? Let’s dive in.
Air Pollution: An Invisible Threat with Tangible Consequences
Air pollution comes in many forms, from the tiny particulate matter (PM2.5 and PM10) that penetrates deep into our lungs to gases like nitrogen oxides (NOx) and carbon monoxide (CO). These pollutants come from a variety of sources, including:
- Transportation: Exhaust fumes from cars and trucks.
- Industry: Emissions from factories and power plants.
- Agriculture: Stubble burning and ammonia from fertilizers.
- Natural Events: Dust storms, wildfires, and volcanic eruptions.
In cities like Delhi, air quality can become so poor that even stepping outside poses severe health risks. This has prompted urgent efforts to predict and improve air quality through innovative technology.
Predicting Pollution with Machine Learning: A Breath of Fresh Air
Traditional methods for predicting air pollution rely on statistical models, but they often fall short when dealing with complex, dynamic systems. Enter machine learning (ML) and deep learning (DL)—tools designed to find patterns in large datasets and make accurate predictions.
How Does It Work?
- Data Collection: Algorithms analyze massive amounts of data, including weather conditions, industrial emissions, and traffic patterns.
- Feature Selection: The models focus on the most relevant factors, such as wind speed, temperature, and pollutant concentration.
- Prediction Models: Advanced methods like neural networks and hybrid models combine spatial and temporal data to forecast pollution levels days in advance.
For example:
- Single Pollutant Models: These predict specific pollutants like PM2.5 using historical data and environmental factors.
- Multi-Pollutant Models: These integrate data from various pollutants to provide a comprehensive air quality forecast.
The Challenges of Clean Air Predictions
While ML and DL models show promise, they aren’t without challenges:
- Data Limitations: Accurate predictions require large, high-quality datasets, which can be difficult to obtain.
- Uncertainty: Rapid changes in weather or human activity can introduce unpredictability.
- Computational Demands: Advanced models require significant computational resources to process complex datasets.
However, researchers are developing hybrid models that combine the strengths of different approaches, achieving better accuracy and interpretability.
Why This Matters: From Personal Health to Planetary Survival
The implications of accurate air pollution prediction are enormous:
Health Benefits
- Early Warnings: Cities can issue alerts to reduce exposure during high-pollution days.
- Policy Development: Governments can target major pollution sources with stricter regulations and incentives for cleaner technologies.
Environmental Impact
- Sustainable Development: Cleaner air supports ecosystems and combats climate change.
- Global Collaboration: Predictive models can inform international efforts to reduce cross-border pollution.
Real-World Success: Innovations in Action
- Delhi’s Air Quality Management: By integrating machine learning with satellite data, officials are better equipped to tackle seasonal smog caused by stubble burning and vehicular emissions.
- Beijing’s Smog Forecasting: Advanced hybrid models predict PM2.5 concentrations, helping residents plan their activities and encouraging cleaner industrial practices.
- Global Early Warning Systems: Countries worldwide are adopting these technologies to monitor air quality, protect vulnerable populations, and design effective policies.
The Future of Air Quality Prediction
Researchers are optimistic about the potential of artificial intelligence to revolutionize air quality management. Emerging technologies aim to:
- Incorporate Satellite Data: Improve spatial resolution for more localized predictions.
- Enhance Real-Time Updates: Use streaming data for instantaneous air quality assessments.
- Develop Early Warning Systems: Create alerts for extreme pollution events, allowing individuals and governments to act quickly.
These innovations promise not only cleaner air but also a more sustainable future.
Let’s Explore Together
Air pollution is a challenge we all share, but science offers hope. What do you think about the potential of technology to solve this global issue? Share your thoughts in the comments or on social media!
- How does air pollution impact your daily life?
- What solutions do you think governments should prioritize?
- How can individuals contribute to cleaner air?
Together, we can turn the tide on air pollution—one breath at a time.
Explore and Learn with Science
Dive into groundbreaking research and inspiring stories with ‘This Week in Science’! Perfect for teachers and science enthusiasts, our free weekly newsletter expands your horizons in teaching and learning. Join us today and reshape your engagement with science. If you liked this blog, please share it! Your referrals help This Week in Science reach new readers.
Leave a Reply