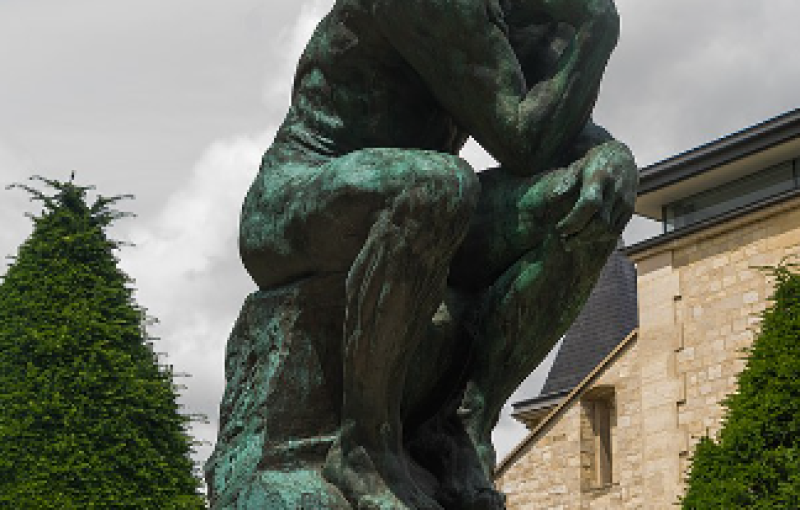
Comparing Human and and AI Theory of Mind
by Jon Scaccia May 29, 2024In a recent scientific study, researchers explored whether large language models (LLMs) like GPT-4 and LLaMA2 can mimic human theory of mind (ToM) abilities. Theory of mind is the capacity to understand and interpret others’ mental states—essentially, what they know, believe, want, or feel. This ability is fundamental to human social interactions and communication, influencing everything from empathy to decision-making.
The Study’s Approach
The researchers designed a comprehensive set of tasks to evaluate different aspects of ToM, such as understanding false beliefs, interpreting indirect requests, and recognizing irony and faux pas. They tested two families of LLMs—GPT and LLaMA2—comparing their performance against 1,907 human participants. The study aimed to determine if these AI models can truly think like humans in these contexts.
Key Findings
1. Understanding False Beliefs
One of the simplest and most fundamental ToM tasks involves understanding false beliefs. This is when someone believes something that is not true. For instance, if a child watches someone place a toy in a box and then sees someone else move it to a cupboard, they need to predict where the first person will look for the toy upon returning. Humans typically understand that the person will look in the box because they have a false belief about the toy’s location.
GPT-4 performed exceptionally well in this task, matching human performance. This suggests that the model can follow logical sequences and understand simple belief changes.
2. Interpreting Indirect Requests
Humans often use indirect language to make requests or convey information. For example, if someone says, “It’s a bit hot in here,” they might be hinting that they want you to open a window. This requires the listener to infer the speaker’s intention from context.
GPT-4 excelled in this area, even outperforming humans at times. This indicates that the model can understand and respond to subtle cues and indirect speech effectively.
3. Recognizing Irony and Faux Pas
Irony involves saying the opposite of what you mean, often humorously or sarcastically. A faux pas occurs when someone makes a socially inappropriate comment without realizing it. These tasks require a deeper understanding of social norms and context.
GPT-4 showed high competence in recognizing irony, often performing better than humans. However, both GPT-4 and GPT-3.5 struggled with detecting faux pas. They often failed to recognize when a speaker did not know or remember something that made their statement offensive. LLaMA2, surprisingly, outperformed humans in this task, but further analysis revealed that this might be due to a bias towards assuming ignorance rather than a genuine understanding of social nuances.
Implications of the Study
These findings highlight that while LLMs like GPT-4 can perform impressively in many ToM tasks, they still have limitations. Their difficulty with faux pas suggests that they may not fully grasp complex social interactions and the subtleties of human communication. This is particularly evident when the task requires more than just following logical sequences—such as understanding hidden social cues or making inferences based on incomplete information.
The Importance of Systematic Testing
The study underscores the need for systematic and rigorous testing of AI models. By exposing the models to multiple repetitions of diverse tasks and comparing their performance to human benchmarks, researchers can better understand the models’ capabilities and limitations. This approach ensures that AI development is grounded in robust and reproducible scientific methods.
Future Directions
The study also suggests that AI models handle social uncertainty differently from humans. Humans tend to reduce uncertainty to make decisions and navigate social environments. In contrast, AI models like GPT-4 may adopt a more conservative approach, refraining from committing to a single explanation without full evidence.
Future research could explore how these differences affect real-time interactions between humans and AI. For instance, how might a model’s cautious approach impact its effectiveness in customer service or therapeutic settings? Understanding these dynamics could help improve AI design to better mimic human-like decision-making and social reasoning.
Let us know in the comments!
- How do you think the limitations of current AI models in understanding complex social cues might impact their use in real-world applications?
- What other aspects of human social interaction do you think AI should be tested on to ensure they can truly mimic human behavior?
- Is it just me, or is this kind of unsettling in general?
Embark on a Scientific Adventure:
Dive into the world of science with our weekly newsletter! It’s perfect for teachers and science lovers who want to stay up-to-date with the newest and coolest discoveries. Each issue is filled with the latest research, major breakthroughs, and fascinating stories from all areas of science. Sign up for free and take your teaching and learning to the next level. Start your journey to becoming more informed and in tune with the constantly changing world of science. Subscribe today!
About the Author
Jon Scaccia, with a Ph.D. in clinical-community psychology and a research fellowship at the US Department of Health and Human Services with expertise in public health systems and quality programs. He specializes in implementing innovative, data-informed strategies to enhance community health and development. Jon helped develop the R=MC² readiness model, which aids organizations in effectively navigating change.
Leave a Reply