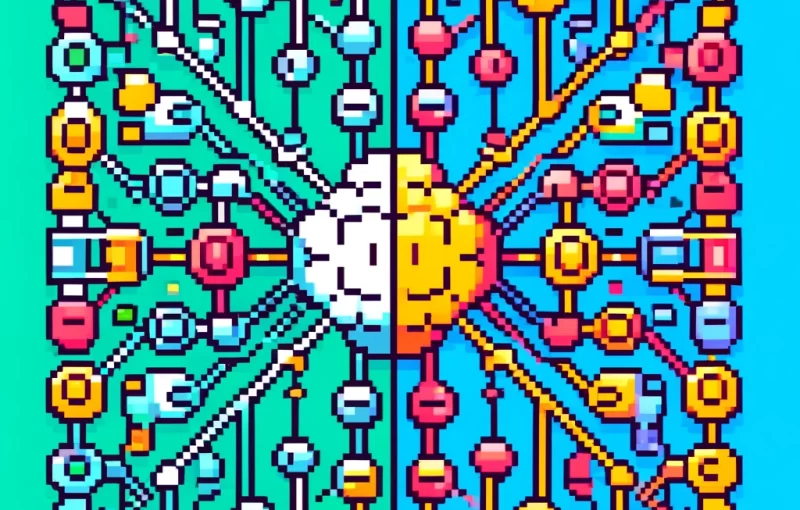
The Potential of Kolmogorov-Arnold Networks
by Jon Scaccia May 6, 2024In the expansive landscape of artificial intelligence, the pursuit of optimizing neural networks is as relentless as it is crucial. The traditional stalwarts of this realm, Multi-Layer Perceptrons (MLPs), though foundational, are not without their limitations—chief among them being their opacity and inefficiency in parameter use. However, a groundbreaking study (not exaggerating) from a team at the Massachusetts Institute of Technology, among others, proposes an innovative alternative: Kolmogorov-Arnold Networks (KANs). This transformative approach not only promises to refine accuracy but also enhances the interpretability of neural networks, a significant stride forward in machine learning.
Understanding the Innovation of KANs
KANs draw inspiration from the Kolmogorov-Arnold representation theorem, a mathematical framework that decomposes complex functions into simpler, one-dimensional components. Unlike MLPs that use fixed activation functions, KANs employ learnable activation functions along the network’s edges. This shift from a node-centric to an edge-centric approach in function application is pivotal. It eliminates the need for linear weight matrices, replacing them with one-dimensional spline functions—flexible mathematical tools that can adapt their shape to fit data more accurately.
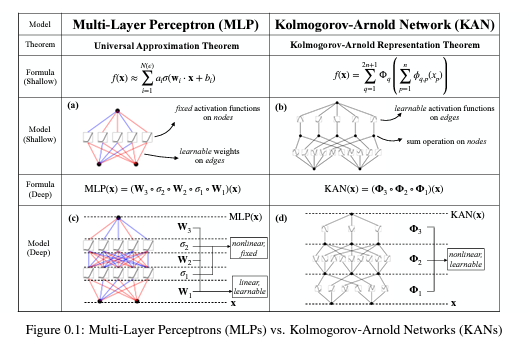
One of the standout benefits of KANs is their efficiency. Where traditional networks might require extensive computational resources, KANs achieve comparable or superior results with significantly fewer parameters. This efficiency is not just theoretical; it has practical implications in solving complex differential equations and data fitting problems, showing remarkable accuracy improvements over MLPs.
The Dual Advantages: Accuracy and Interpretability
Accuracy in machine learning is often a trade-off with interpretability. Yet, KANs manage to bridge this divide gracefully. By leveraging the intrinsic properties of splines, which are adept at modeling low-dimensional data, KANs effectively tackle the curse of dimensionality—a notorious challenge where the performance of data analysis tools degrades as the dimensionality of the data increases. Moreover, the structure of KANs allows for clearer insights into the decision-making processes of the network, making them not just tools for prediction but also for understanding.
The practical applications of such an advancement are vast. In fields where precision and explanation are paramount—such as autonomous driving, financial modeling, and personalized medicine—KANs could provide the necessary edge in predictive performance while also furnishing clear, interpretable models that can be trusted and easily scrutinized.
Collaborative and Educational Potential
The implications of KANs extend beyond mere computational improvements. Their architecture, which simplifies complex models into understandable components, offers a unique educational tool for students and professionals eager to understand the underlying mechanics of neural networks. Furthermore, the adaptability of KANs makes them ideal collaborators in scientific research, where they can aid in the discovery and verification of new scientific laws.
Looking Forward
The journey of KANs from a theoretical construct to a standard tool in AI applications may still be in its early stages, but the path is promising. Future research will undoubtedly focus on refining these networks, improving their scalability, and exploring their full potential across various domains.
The advent of Kolmogorov-Arnold Networks marks a significant milestone in the evolution of neural network design, heralding a future where AI is not only powerful and efficient but also transparent and interpretable. As we stand on the brink of this new era, the anticipation of what comes next in AI is palpable and thrilling.
Engage with Us
Do you find the concept of KANs as fascinating as we do? Have thoughts on how this might change the technology landscape, or questions about the technical specifics? Share your insights in the comments below, and let’s explore the future of AI together!
Unlock the Secrets of Science:
Get ready to unlock the secrets of science with ‘This Week in Science’! Our newsletter, designed specifically for educators and science aficionados, delivers a weekly digest of revolutionary research, innovative discoveries, and motivational tales from the scientific frontier. Subscribing is your key to a treasure trove of insights that can revolutionize your approach to teaching and learning science. Sign up today at no cost and start a journey that deepens your understanding and passion for science.
About the Author
Jon Scaccia, with a Ph.D. in clinical-community psychology and a research fellowship at the US Department of Health and Human Services with expertise in public health systems and quality programs. He specializes in implementing innovative, data-informed strategies to enhance community health and development. Jon helped develop the R=MC² readiness model, which aids organizations in effectively navigating change.
Leave a Reply