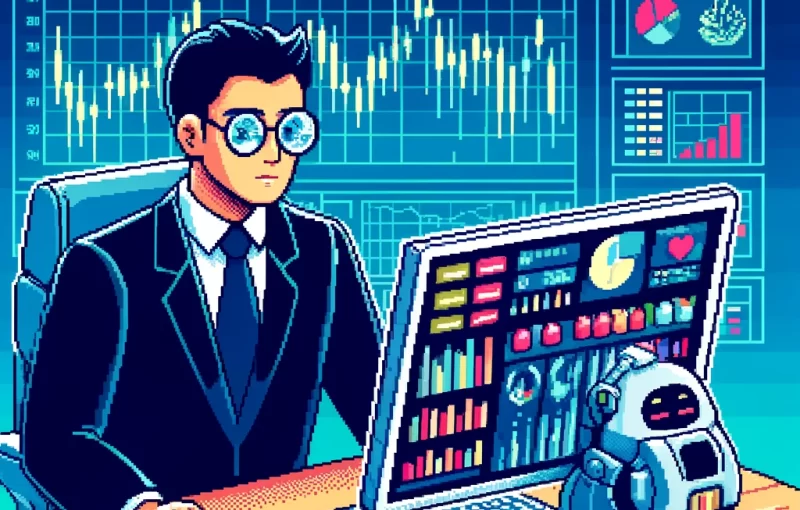
AI Revolution in Investment Portfolio Management
by Jon Scaccia June 11, 2024Artificial intelligence (AI) has revolutionized many industries, and the financial sector is no exception. One area where AI has made significant strides is investment portfolio management. Portfolio management’s primary goal is to balance risk and reward by optimally allocating assets like stocks, bonds, and cash. Traditionally, this process involves three steps: planning, execution, and feedback. Each step has its objectives and methods, and recent advancements in AI have transformed how these tasks are performed.
Disclaimer: This is not financial advice! This is just about how AI is informing financial asset management! If you want advice, talk to someone like Envest.
The Traditional Portfolio Management Process
- Planning: The first step involves setting up an investment policy based on the client’s needs, circumstances, and constraints. This policy includes strategic asset allocation (SAA), which defines the upper and lower boundaries for asset class allocation, risk tolerance, and risk capacity.
- Execution: This step starts with analyzing macroeconomic conditions across countries and asset classes to determine capital allocation. Security analysis follows, enabling the selection of individual securities within each asset class to construct the overall portfolio.
- Feedback: After the investment period, the portfolio’s performance is evaluated, market conditions are updated, and adjustments are made to the investment policy if necessary. The portfolio is then rebalanced to align with the updated policy.
From Markowitz to AI
Since Harry Markowitz introduced the mean-variance model in 1952, various frameworks have evolved to address the complexities of portfolio management. The model’s main idea is to select investments based on their collective contribution to the overall portfolio’s risk and return, rather than on individual merits. However, the dynamic nature of financial markets, globalization, and new risks like geopolitics and systemic risk have made portfolio management increasingly challenging.
AI in Portfolio Management
AI techniques have dramatically improved portfolio management, enhancing traditional methods and creating new opportunities for better returns. Here’s how AI is making a difference:
- Machine Learning (ML): ML algorithms can predict asset prices by learning from historical data. For instance, reinforcement learning (RL) is promising for dynamic portfolio optimization, and text mining and sentiment analysis can incorporate real-time news into portfolio strategies.
- Deep Learning (DL): DL models can optimize portfolios directly or mimic index funds with fewer assets. These models can process vast amounts of data, identify complex patterns, and improve risk management.
- Dimensionality Reduction: Techniques like Principal Component Analysis (PCA) can detect latent factors in asset prices, improving diversification.
Challenges and the Role of Explainable AI (XAI)
AI-based portfolio management often relies on complex, black-box models, which can be difficult to explain. This lack of transparency raises issues of trust among stakeholders such as investors and regulators. To address this, explainable AI (XAI) methods are being developed to make AI decisions more understandable. These methods ensure that AI models are transparent, fair, and accountable, fostering trust and enabling wider adoption.
Regulatory Developments
Recent regulatory changes in the European investment business highlight the need for transparency in AI applications. Explainable AI can help meet these requirements, providing clarity on how AI models make decisions and ensuring compliance with regulatory standards.
What do you think?
- How do you think AI will further revolutionize portfolio management in the next decade?
- What are your thoughts on the balance between AI model complexity and the need for transparency in financial decision-making?
Conclusion
AI is transforming investment portfolio management by enhancing decision-making, improving risk management, and increasing operational efficiency. However, the complexity of AI models necessitates the development of explainable AI techniques to ensure transparency and build trust among stakeholders. As regulatory frameworks evolve, the financial industry must adopt these advancements to maintain ethical standards and ensure accountability.
Unlock Science Secrets
Discover revolutionary research and innovative discoveries with ‘This Week in Science’! Designed for educators and science lovers, our free weekly newsletter offers insights that can transform your approach to science. Sign up now and deepen your understanding and passion for science.
About the Author
Jon Scaccia, with a Ph.D. in clinical-community psychology and a research fellowship at the US Department of Health and Human Services with expertise in public health systems and quality programs. He specializes in implementing innovative, data-informed strategies to enhance community health and development. Jon helped develop the R=MC² readiness model, which aids organizations in effectively navigating change.
Leave a Reply